Statistical Description of Turbulent Fluid Flow in a Pipe
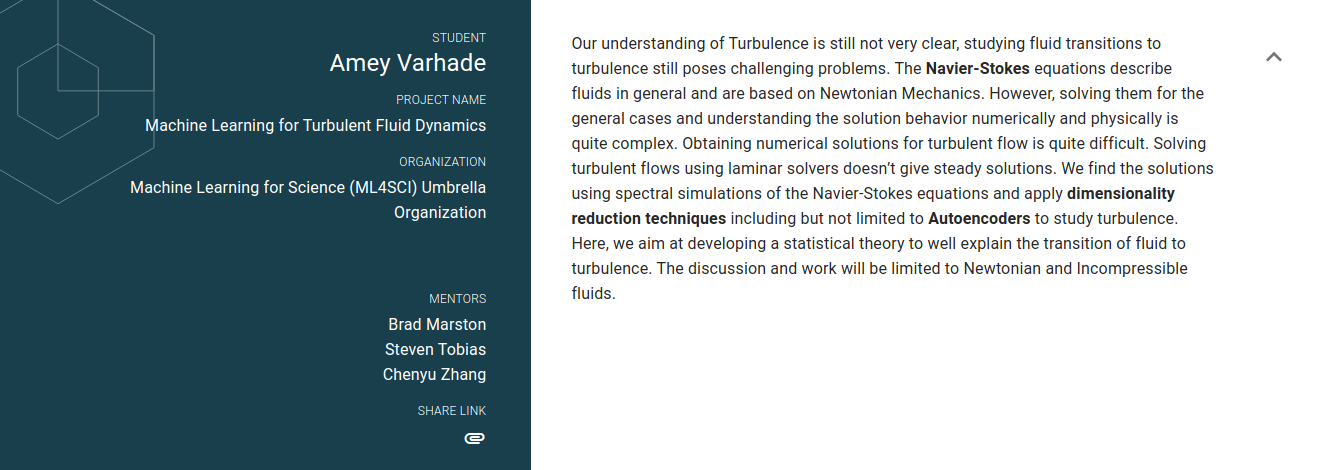
Project Description, ML4SCI
One of the most important outstanding questions in fluid dynamics is the study of how and why uniform flow transitions to chaotic motion, or turbulence. Turbulent flow is a vital aspect in the design of airplanes, cars, and boats, but also in climate science and chemical engineering. Unsupervised machine learning will be used to find coherent structures in simulated flow in a pipe. Results will be used to develop a statistical theory of the transition to turbulence. Apply dimensionality reduction techniques to a dataset of fluid simulations, such as proper orthogonal decomposition (POD), principal component analysis (PCA), or autoencoder neural networks. Determine the most important structures in predicting the onset of turbulence.
Project Synopsis
Our understanding of Turbulence is still not very clear, studying fluid transitions to turbulence still poses challenging problems. The Navier-Stokes equations describe fluids in general and are based on Newtonian Mechanics. However, solving them for the general cases and understanding the solution behavior numerically and physically is quite complex. Obtaining numerical solutions for turbulent flow is quite difficult. Solving turbulent flows using laminar solvers doesn’t give steady solutions. We find the solutions using spectral simulations of the Navier-Stokes equations and apply dimensionality reduction techniques including but not limited to Autoencoders to study turbulence. Here, we aim at developing a statistical theory to well explain the transition of fluid to turbulence. The discussion and work will be limited to Newtonian and Incompressible fluids.